The impact of machine learning on education accessibility
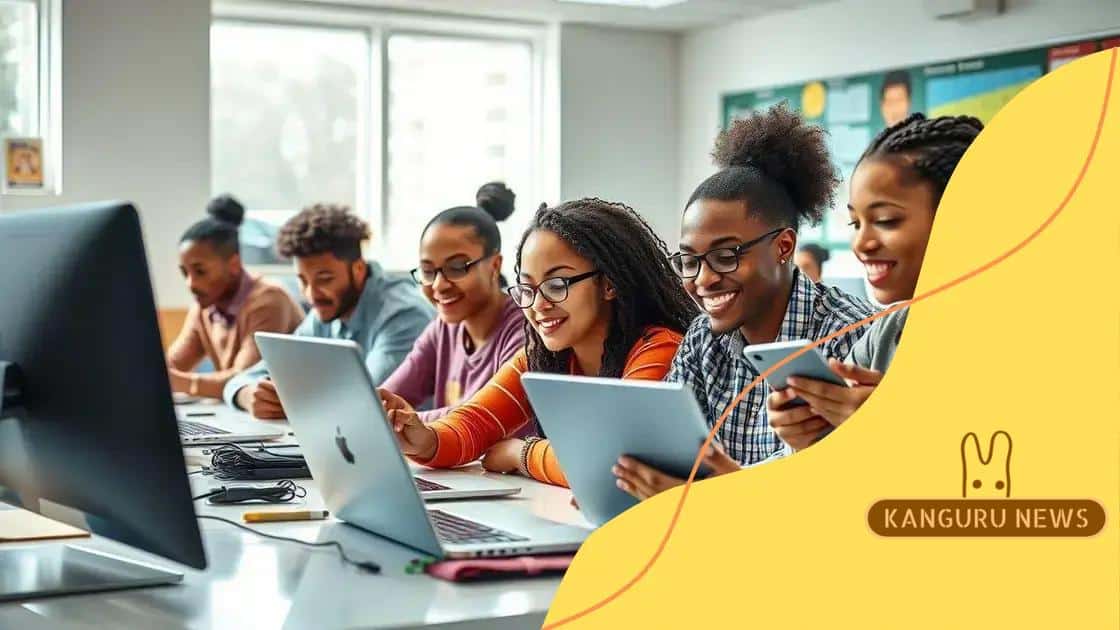
The impact of machine learning on education accessibility enhances personalized learning experiences, improves administrative efficiency, and fosters inclusive environments for diverse student needs.
The impact of machine learning on education accessibility is changing the way students learn, making education more inclusive and tailored to individual needs. Have you ever wondered how advanced algorithms can provide personalized learning experiences?
Understanding machine learning in education
Understanding machine learning in education is essential for recognizing its potential to enhance teaching and learning. This technology has become a powerful tool that helps in creating more personalized learning experiences.
What is Machine Learning?
Machine learning refers to the use of algorithms that allow computers to learn from data. This means that machines can identify patterns and make decisions based on past information.
Benefits in Education
By applying machine learning in educational settings, institutions can improve student outcomes in several ways:
- Personalized learning paths for each student.
- Early identification of students who may need additional support.
- Real-time feedback for both students and teachers.
The power of machine learning lies in its ability to adapt to individual learning preferences. This can make education more engaging and effective, as each student receives the right resources based on their unique needs.
How Does It Work?
Machine learning algorithms analyze vast amounts of data from various sources, including test scores and student interactions with learning materials. This data helps educators understand trends and individual learning styles, enabling tailored instructional methods.
Furthermore, as these systems continue to learn from new data, they become even more accurate and relevant over time. The integration of machine learning in education makes it possible to create immersive and interactive learning experiences for students.
Overall, understanding machine learning in education can be a game changer. By leveraging this technology, schools can provide more equitable access to quality education, ensuring that every student has the opportunity to succeed.
How machine learning personalizes learning experiences
Machine learning significantly personalizes learning experiences for students, making education more effective and engaging. By analyzing data, machine learning can identify individual strengths and weaknesses.
Adaptive Learning Systems
Adaptive learning systems are designed to adjust content based on student performance. These systems provide:
- Customized learning paths tailored to each student.
- Instant feedback that helps learners understand their progress.
- Content recommendations based on prior interactions.
Through this process, students receive lessons that cater to their unique learning styles, enhancing their understanding of the subject matter.
Data-Driven Insights
Machine learning algorithms gather data from various sources, allowing educators to track progress effectively. Teachers can make informed decisions based on:
- Student engagement levels during different activities.
- Common misconceptions shared by the class.
- Areas where students excel or struggle.
This data allows for more targeted instruction and helps educators provide necessary interventions when needed.
As students continue learning, machine learning systems refine their approaches over time, enabling even greater personalization. This constant adaptation helps ensure that every student feels supported in their educational journey.
Ultimately, the integration of machine learning into educational practices not only fosters a deeper understanding but also boosts students’ confidence and motivation.
Challenges in implementing machine learning
Implementing machine learning in education brings many exciting opportunities, but it also comes with several challenges. Understanding these hurdles is important for educators and administrators.
Data Privacy Concerns
One major challenge involves data privacy. Schools must ensure that student data is protected. This includes:
- Safeguarding personal information from breaches.
- Complying with regulations regarding data use.
- Ensuring students and parents are informed about data collection.
Without proper measures, schools risk losing student trust, which is essential for a successful learning environment.
Integration with Existing Systems
Another issue is integrating machine learning systems with existing educational technologies. Many schools rely on a variety of tools and platforms that may not easily connect. This can lead to:
- Increased costs for new software and training.
- Disruption in current workflows.
- Frustration among teachers and students.
To tackle this, schools must evaluate their current systems and plan for gradual integration.
Need for Training and Support
Effective implementation also requires proper training for educators. Teachers need support to understand how to use machine learning tools effectively. This includes:
- Training sessions on new technologies.
- Resources to help troubleshoot common problems.
- Ongoing support from administrators.
Without adequate training, teachers may struggle to use these tools, limiting the potential benefits for students.
Overall, addressing these challenges is crucial for a successful transition into machine learning in education. By focusing on data privacy, integration, and teacher training, schools can make this transition smoother.
Real-world examples of machine learning in education
Real-world examples of machine learning in education highlight how technology can transform learning experiences. Schools and educational platforms are leveraging machine learning to create effective solutions that address diverse student needs.
Personalized Learning Platforms
Many platforms now use machine learning algorithms to tailor education to individual students. For instance:
- Systems analyze student performance over time.
- They adapt learning material based on student strengths and weaknesses.
- Students receive recommendations for further readings or exercises suited to their learning pace.
This personalization helps students grasp complex concepts by providing resources that match their learning styles.
Intelligent Tutoring Systems
Intelligent tutoring systems are another excellent example. These systems act like personal tutors that offer:
- Immediate feedback on exercises and quizzes.
- Customizable learning paths tailored to student needs.
- Targeted practice on specific subjects where students struggle.
Such systems enhance learning by making education more interactive and responsive.
Administrative Efficiency
Implementing machine learning isn’t limited to the classroom. Educational institutions also use it for administrative tasks. They can:
- Analyze enrollment trends to optimize resource allocation.
- Predict student enrollment patterns to plan accordingly.
- Improve communication strategies by understanding student engagement metrics.
This data-driven approach helps educational institutions become more efficient in managing their resources and better supporting their students.
By looking at these real-world examples, it becomes clear that machine learning in education offers numerous advantages, making learning more engaging, efficient, and accessible to all.
Future trends in educational technology and accessibility
Future trends in educational technology promise to bring exciting changes, especially regarding accessibility. The goal is to create learning environments that cater to diverse needs and abilities.
Increased Use of Artificial Intelligence
Artificial Intelligence (AI) will play a key role in shaping educational technology. Students will benefit from personalized learning experiences that adapt to their specific needs. For example:
- AI can analyze student data to create customized lesson plans.
- It enables real-time feedback on student performance.
- AI-powered tools will help identify areas where students struggle and offer targeted assistance.
This adaptability ensures no student gets left behind.
Virtual and Augmented Reality
Virtual Reality (VR) and Augmented Reality (AR) will enhance hands-on learning experiences. These technologies make complex subjects more accessible and engaging. Some advantages include:
- Immersive environments that provide interactive learning opportunities.
- Opportunities for students with disabilities to experience learning in unique ways.
- Realistic simulations that can complement traditional teaching methods.
As these technologies advance, schools can offer more inclusive learning experiences for all students.
Focus on Collaborative Learning
The rise of online learning platforms will promote collaborative experiences among students. Tools will enable groups to work together seamlessly, regardless of their location. This trend emphasizes:
- Better communication and collaboration skills.
- Access to resources and knowledge-sharing among peers.
- Flexibility in how and when students learn.
As education evolves, fostering collaboration will be essential for preparing students for the global workforce.
In summary, the future of educational technology holds exciting opportunities for promoting accessibility and ensuring that every student has the tools they need to succeed. By integrating AI, VR, AR, and collaborative learning, education will become more inclusive and effective.
In conclusion, the impact of machine learning on education accessibility is profound and transformative. As we move forward, integrating innovative technologies like Artificial Intelligence, VR, and collaborative platforms will create learning environments that cater to all students. By focusing on these future trends, educators can ensure that every learner has equal access to quality education. The possibilities for enhancing teaching and learning are limitless, paving the way for a more inclusive and effective educational landscape. Let’s embrace these changes and work together to unlock the full potential of education for everyone.
FAQ – Questions Frequently Asked About Machine Learning in Education
How does machine learning personalize learning experiences?
Machine learning analyzes student data to create customized learning paths and provide targeted feedback, making education more effective.
What are some examples of machine learning in education?
Examples include personalized learning platforms, intelligent tutoring systems, and administrative tools that help manage student data.
What challenges do schools face when implementing machine learning?
Challenges include data privacy concerns, integration with existing systems, and the need for adequate training for educators.
What trends should we expect in educational technology?
Future trends include increased use of AI, virtual and augmented reality for immersive learning, and a focus on collaborative learning environments.